What Happens When Audit Meets AI?
New research on artificial intelligence in audit examines the impact when human auditors trust—or don’t trust—the machines.
By Joshua Herbold, Ph.D., CPA | Fall 2023
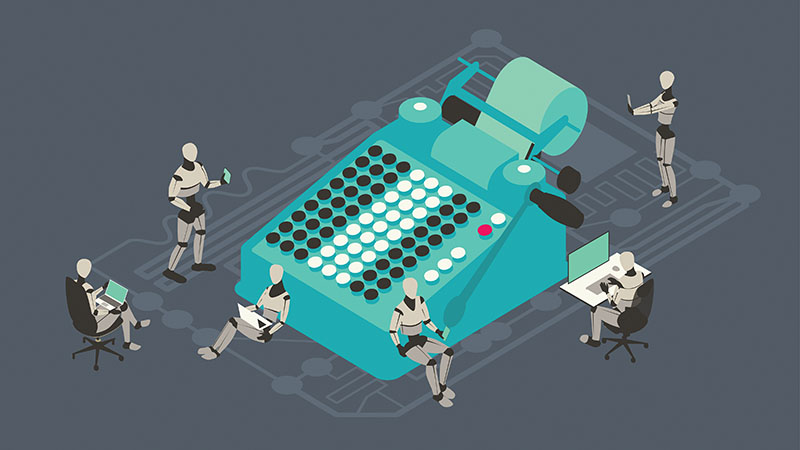
Since at least the Industrial Revolution, humans have been reluctant to fully trust machines. From John Henry’s race against a steam-powered hammer, to Neo’s rebellion against the Matrix, our folklore warns of the dangers of creating inventions that are faster, better, and smarter than their creators. But does that really apply to the accounting and finance world?
“It’s great that firms are spending time and resources to develop artificial intelligence (AI) systems that can help accounting and finance professionals, but it’s going to be a problem if auditors and accountants don’t want to use it,” cautions Jenny Ulla, Ph.D., CPA, an assistant professor of accountancy in the Gies College of Business at the University of Illinois Urbana-Champaign.
Large professional services firms are already ramping up substantial investments in technologies based on AI, machine learning, generative pre-trained transformers (GPT), and large language models—the underlying programming behind products like OpenAI’s ChatGPT and Google’s Bard. In one of the largest such investments to date, KPMG has committed to invest $2 billion in Microsoft’s generative AI technology and expects this investment to lead to over $12 billion in incremental revenues over the next few years.
But as firms explore the potential for these new technologies, regulators have expressed concern about overreliance on them. The Public Company Accounting Oversight Board’s Standards and Emerging Issues Advisory Group recently announced that AI is one of its top three concerns and held an open meeting to “consider proposing for public comment amendments to existing standards addressing aspects of designing and performing audit procedures that involve technology-assisted analysis of information in electronic form.” United States Securities and Exchange Commission Chair Gary Gensler has even said that overreliance on AI by financial institutions could end up being the cause of the next financial crisis.
While regulators worry about overreliance on AI, new research from Ulla and her co-authors examines the opposite. In “Man Versus Machine: Complex Estimates and Auditor Reliance on Artificial Intelligence,” Ulla and researchers Benjamin Commerford, Sean Dennis, and Jennifer Joe explore the phenomenon known as “algorithm aversion,” which could lead to underreliance on the technology in which firms are investing so heavily. If users underrely on technology, the shift to AI may not yield the anticipated improvements in audit quality, for instance, especially when complex, subjective estimates are involved.
Algorithm aversion occurs when humans trust input and advice from other humans more readily than from algorithms, even when the advice and the underlying situation are the same.
“Do people treat estimates that come from a human versus an AI system differently?” Ulla asks. “Algorithm aversion is the tendency to discount computer-based advice more heavily than human advice. It’s been shown in a whole range of decision-making scenarios, from movie recommendations, to dating advice, to forecasting stock prices. Part of it could be that decision makers don’t understand how the systems work, and part of it could be that they don’t trust that the AI systems are well-suited to the tasks. The research on algorithm aversion finds that people tend to believe that computers are best suited for simple or objective tasks, and that computer systems can’t think or adapt in real time.”
Ulla’s research suggests that auditors may be reluctant to rely on AI-generated advice, even if it’s more accurate than human advice. Ulla and her co-authors examined whether auditors evaluating complex accounting estimates would be susceptible to algorithm aversion: “Participants in our study completed a scenario where an audit task involved a potential audit adjustment. The client was a bank, and the client’s stance was that no adjustment was needed to their allowance for loan loss (ALL). Participants were given a report from their firm’s specialist that contradicted management’s evidence and suggested that their ALL was materially understated.”
Although all participants saw the same firm-provided report, some participants were told that the report came from the firm’s in-house valuation group (i.e., humans), while others were told that the report was from the firm’s proprietary AI system. Regardless of the source, identical language was used to describe the accuracy and reliability of the report.
Participants in both conditions proposed audit adjustments to increase the client’s ALL. On average, however, participants who believed the firm evidence came from the firm’s proprietary AI system proposed ALL adjustments in dollar amounts that were 23% lower than participants who thought the evidence was provided by humans, a result that’s consistent with algorithm aversion.
When the nature of the evidence provided by the client was more objective, the difference in proposed audit adjustments grew even larger. Ulla and her co-authors also varied the banking client’s underlying information used to develop their conclusion that no adjustment was necessary to the ALL.
As described in the paper, some study participants were told that the client “relies heavily on the judgment of loan officers and credit analysts, who use a variety of methods and information (e.g., discussions with real estate brokers) to develop estimates for a key parameter in the ALL estimate (i.e., collateral values).” This information is considered relatively more subjective. Other participants were told that the client “relies on client-selected, detailed market data (i.e., real estate price indices) to update collateral values in a standardized manner.” This method is considered relatively more objective.
The results? The effects of algorithm aversion became even clearer: For participants who believed that the client’s evidence was more objective, seeing an AI-based audit firm report (instead of a human-based report) led to a 43% lower proposed audit adjustment dollar amounts.
Ulla describes it this way: “Auditors were more than willing to rely on AI-based evidence from their firm and propose audit adjustments based on that evidence. But they were quick to discount an AI system when the client’s evidence seemed to be relatively more objective in nature. If there was any doubt present, participants were willing to discount the AI system.”
Does this mean that firms shouldn’t use these new technologies? “Not at all,” Ulla says. “However, it’s difficult to predict who’ll use AI or not, because every situation is different, and it’s a very nuanced area. It depends on the person, the task, and the scenario. It could even depend on the seniority of the decision maker. It gets even more complicated if the client is using AI as well.”
This awareness is a central theme for much of Ulla’s research. “I struggled so often as an auditor, and now I wish I would’ve read some of the research that was out there,” she admits. “It would’ve helped so much to see where some of the pitfalls and blind spots were.”
What’s next in this line of research? Ulla says, “Another paper we’re working on examines how auditors react to misestimates committed by humans versus AI systems. Our preliminary results show that if an AI system makes a mistake, people immediately abandon the system and say, ‘I don’t want to use that.’ But if a human makes a mistake, they’re more willing to give the human the benefit of the doubt.”
Ulla’s advice for firms investing in AI-driven technologies is to consider the human element: “The main takeaway is awareness. Firms should be aware that algorithm aversion could happen, and it could have a significant effect on the quality of your services. So, firms should be sure to include that awareness in their development of, and training on, these technologies. An auditor might not fully understand how the AI system made its conclusion, but they still have to go to the client and explain it. That puts the auditor in a very difficult spot. But, if we can cautiously embrace these new technologies, this will be an exciting time for our profession.”
Joshua Herbold, Ph.D., CPA, is a teaching professor of accountancy and associate head in the Gies College of Business at the University of Illinois Urbana-Champaign and sits on the Illinois CPA Society Board of Directors.
Related Content: